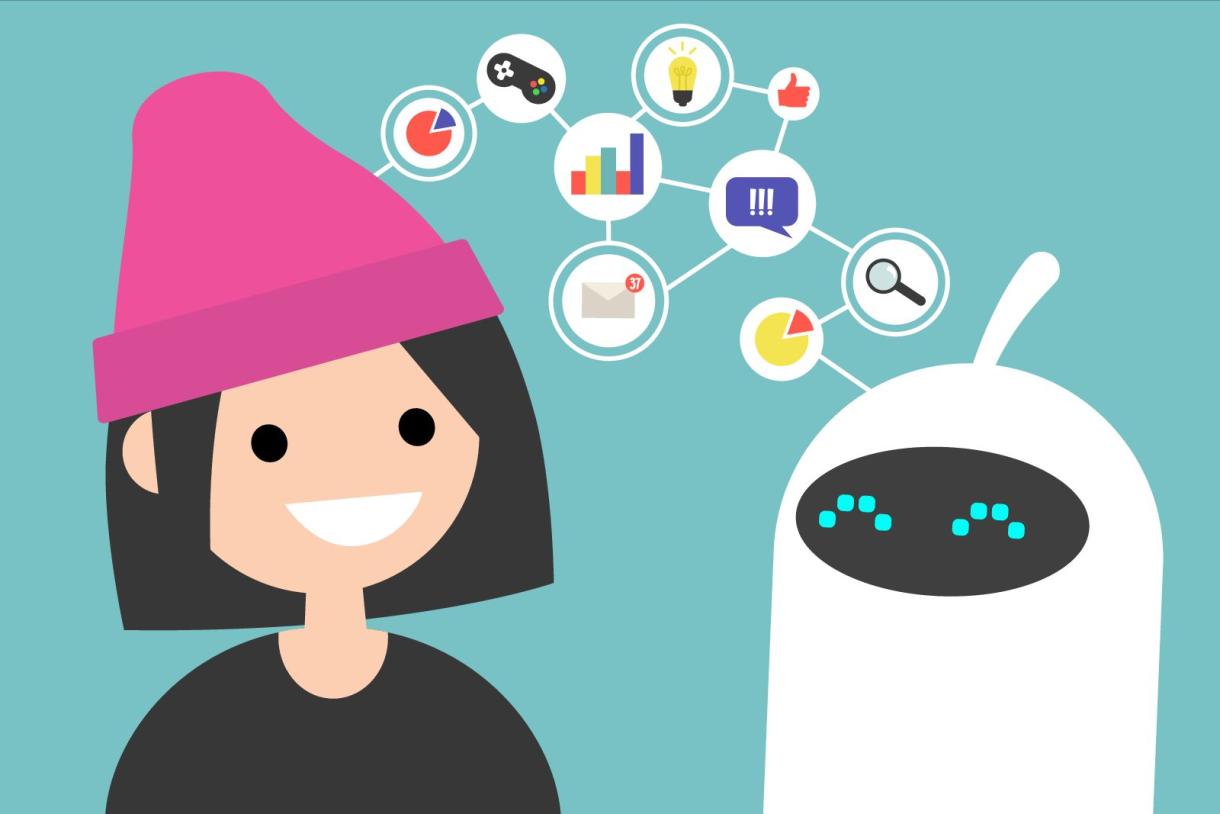
이 글의 초고는 제6회 인공지능인문학국제학술대회(ICAIH2023)에서 발표되었으며, 이 글은 학술대회 후원기관이었던 Heinrich Böll Stiftung Hong Kong의 essay collection "Living with Machines: Communications and Gender in AI and Robotics"에 먼저 기고되었습니다. Alarming Under-representation The figures provided – 20%, 18%, 2.5 %, and 1.4% – highlight a profound crisis within the economic and academic sphere of artificial intelligence (AI). It is observed that 20% of Latinx programmers earn less on average than their white male counterparts in the US (p.13). Only 20% of professors in the field of AI are women. Only 18% of the authors of the 21 most important conferences in this field are women. A mere 2.5% of the permanent employees at the tech company Google are black. Furthermore, within associations advocating for the common good use of artificial intelligence, only 1.4% of the activists are Black (p.30). These statistics collectively reflect a profound issue within the realm of Artificial Intelligence. Timnit Gebru, the former head of Google's ethical AI department, has referred to this lack of diversity among AI developers as a "diversity crisis". This crisis is compounded by insufficient or obscure data. The limited data available concerning corporations, predominately from US companies, are distorted. For instance, Google’s figures only encompass 80% of their employees, specifically excluding those departments composed entirely of white men. Additionally, corporations such as Tesla or Netflix do not disclose diversity figures. Consequently, certain demographic groups are completely excluded from gender-related surveys. Individuals who identify outside the female-male binary are not accounted for in the cited figures. Moreover, it is questionable whether the women and men represented in these studies are cisgender or transgender individuals (p.4). Moreover, this diversity crisis extends beyond representation and permeates to AI products themselves. In 2018, Amazon used software for candidate selection in application process, which was designed using datasets predominantly comprised of successful applications from men. Consequently, the software exhibited a bias favouring male applicants (p.28). However, what are the reasons behind these discriminatory structures? Since the 1970s, a widely accepted thesis, particularly in political and academic circles, has been that there is a scarcity of marginalised individuals, particularly women, in the IT field. Policy measures aimed at fostering inclusivity have largely been based on this perspective, such as initiatives like Girls Day in Germany or targeted promotion programs for women in STEM fields. Regrettably, these approaches have yielded limited success in practice. For example, the representation of women in STEM subjects in Germany has remained stagnant for years, with only marginal growth typically occurring, often by a few percentage points. Therefore, in this article, I would like to offer a fresh perspective on the issue. I propose the thesis that marginalised groups, including women and people of colour, are already involved in AI-related work, albeit predominantly in precarious positions without decision-making power, rather than in top positions. I will delve into three aspects of AI production: the initial generation of knowledge at universities as the foundation, the production of AI and its hardware as a catalyst for global labour inequalities, and the realm of crowd work as a precarious field associated with AI. Gendered and Racialised Dynamics in AI Work I would like to assert that working on and with AI is inherently influenced by gender and race. As early as the 1990s, researchers began highlighting the distinct gendered production sphere of artificial intelligence, particularly within the university setting, which resulted in (a) the invisibility of numerous AI-related activities and (b) the exclusion of women. In one of the pioneering ethnographic studies on AI research, the anthropologist Diana Forsythe revealed that only programming, but not other aspects like collaborative exchanges in team meetings, was recognised as AI work by those involved (pp. 33-34). Additionally, scholars such as Lucy Suchman (p.200-205) and Alison Adams (p.45-46) have drawn attention to the specific exclusion of women in university contexts, including instances of sexual abuse. Therefore, I propose that we acknowledge the gendered and racialized nature of work on and with AI. To do so, we must first define the concept of division of labour in relation to AI.
Drawing on Shoshana Zuboff’s perspective, which extends the concept of division of labour to encompass work with new digital technologies, I understand division of labour as a division of knowledge. Zuboff argues that division of labour, once associated with the transition from feudal to Fordist societies, no longer solely shapes the idea of commodity production but also plays a role in the social order itself (p.50-51). Furthermore, previous studies examining gendered dynamics within the field of AI have highlighted the exclusion of women within academic settings. For example, anthropologist Diana Forsythe and media theorist Gertraud Koch have demonstrated how sexist work environments have marginalised women in their respective work contexts in Germany and the US. Forsythe attributes the exclusion of women in AI research labs to the devaluation of their skills in comparison to their male colleagues, the construction of women as "others" by male-dominated research groups, and the invisibility of certain tasks associated with femininity, such as secretarial work (cf. Forsythe 2002, pp. 169-173). In a similar vein, Sarah Myers West, in a study on fairness and AI based on MIT research from the 1980s, highlights that "many women are treated as if they were invisible in technical situations... [they are] overlooked in technical discussions and excluded from group efforts" (p.11). Global Production and Labour Exploitation in AI Hardware The impact of AI extends beyond academic settings to the realm of hardware, involving the devices to run AI programs. Donna Haraway, dating back to the early 1990s, highlighted the resource-intensiveness of computer technology and its deceptive "transparent" appearance (p.39). She drew attention to the fact that the hardware powering programs, especially AI, originated from the Global South and was manufactured under exploitative labour conditions by women of colour (p.54-55). Examining the life cycle of modern smart devices, media scientist Jennifer Gabrys explains the prevalent disposable nature of contemporary technologies. Once used, modern mobile phones, computers, and tablets become compatible with the latest software developments, rendering them non-portable for further use over the years (p.2). The disposable approach to hardware drives the need for continuous (p.68-71) development and utilization of technologies such as artificial intelligence. Gabrys highlights the global recycling chain and the predominant role of the Global South in recycling these devices to produce resources for the next generation of smart devices. Unfortunately, this process occurs under precarious and dangerous conditions, jeopardizing the lives of workers who are often exploited through low wages (p.29). Haraway further examines the assembly of increasingly smaller technical hardware, particularly microchips, and exposes the racialised and gendered dimensions underlying this labour. She argues that this miniaturised work is outsourced to women from the Global South (p.55-57). Crowd Work in AI and the Global South The Global South plays a significant role in AI production, particularly in so-called crowd work. On various platforms, workers offer their services as self-employed individuals, engaging in micro-work orders that can be completed within minutes and paid out in minimal amounts (p.7).
Universities capitalize on these resources to reduce the number of permanent jobs attached to the institution. This allows them to undertake large-scale projects involving automated classifications and other AI releated tasks within a short timeframe, while benefitting from cost-effective labour. Anthropologists Mary Gray and Siddharth Suri describe this phenomenon in their study on crowd work (p. xvii). The authors present a nuanced picture on the gender division and global perspectives within this field. Crowd work is not exclusively female-based or associated with femininity. Instead, according to the authors, gender differences in work choices are influenced by diverse justifications, which, in turn, vary significantly based on global location. However, the researchers note that individuals engaging in crowd work are often highly educated, with approximately 80% of their panel respondents holding at least a bachelor's degree (p.29). They also discovered that women who undertake such tasks, especially those from the US, value the advantage of working from home which allows them to balance care responsibilities and other commitments. In contrast, women from India perceive crowd work as a form of independent labour and an avenue for emancipation, offering an opportunity distinct from traditional care work within family contexts. However, a clear gender divide persists in terms of well-paid jobs in the development of intelligent software (p.29). Although work in the field of AI can be diverse in terms of gender or race, such diversity often goes unrecognised. While there is an ample presence of crowd workers from the Global South, the representation of professors from the same regions at Ivy League universities remains inadequate. Consequently, these emergent fields of work continue to be entrenched in a gendered divide where statistically white US cisgender men reap financial benefits, while a growing number of individuals experience precarious employment (cf. West 2020) (p.71). Conclusion Understanding the present discriminating structures is the first step to social change. The exploration of AI's global dimensions of division of labour reveals intricate layers of power dynamics, exploitation, and gender inequalities. From the production of AI hardware in the Global South to the prevalence of crowd work platforms, these issues permeate the entire ecosystem of AI. Efforts must be made to address the biases and injustices that persist in AI algorithms, data, and workforce composition. Creating a more inclusive and diverse landscape in AI requires dismantling the barriers that prevent underrepresented groups, particularly from the Global South, from accessing prestigious academic positions and well-paid roles in software development. By embracing and prioritizing fair labor practices, we can shape an AI landscape that is more just, equitable, and reflective of the diverse world it serves. Revision of an article first published online in The Irish Times, 4/1/ 2023. This article is part of the essay collection "Living with Machines: Communications and Gender in AI and Robotics" published by Heinrich Böll Stiftung Hong Kong in Aug-Sep 2023, as post-conference publishing project of the 6th International Conference on Artificial Intelligence Humanities (ICAIH) 2023 hosted by Humanities Research Institute at Chung-Ang University. Katharina Klappheck(Head of Feminist Internet Policy, Gunda Werner Institute)
|